Here comes the sun: Is machine learning about to transform our world?
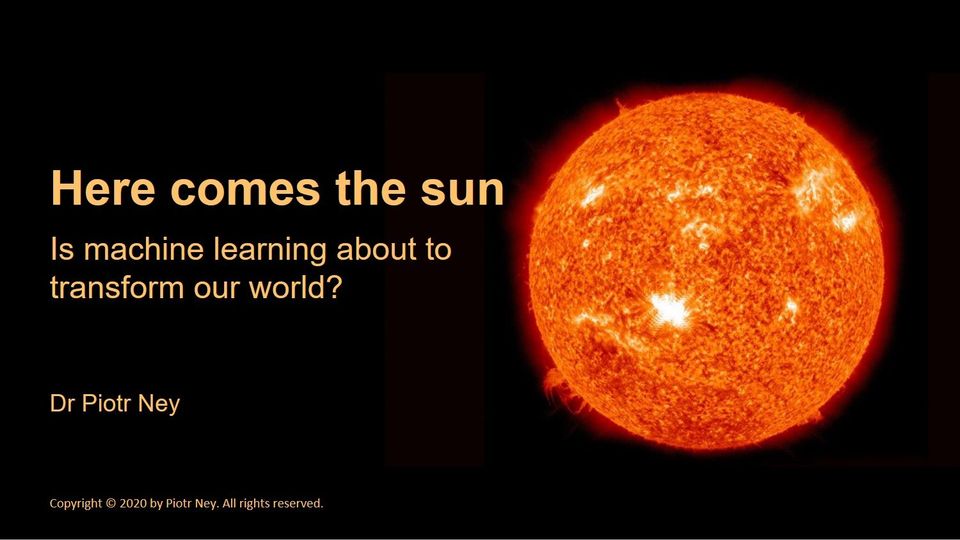
[London, 17 June 2020]
The promise of controlled thermonuclear fusion replacing fossil fuels and fission to power the world has eluded us for decades. An artificial, compact sun here on earth to give us limitless, safe and clean energy, leaving no carbon dioxide or radioactive waste in its wake.
I last worked in nuclear physics research some thirty eight years ago. Since then, I have delivered many innovation and radical transformation projects in business, lately often driven by digital enablers such as artificial intelligence (AI) systems. Claims processing in insurance. Risk management in banking. Hyperpersonalisation of convergent offers in media and telecomm industries. Through these and many other applications of AI, I have helped to improve customer and colleague experience, product innovation and the profitability of companies.
But the true global impact of AI lies beyond making some profitable companies even more profitable. It is proving to be a key weapon in our existential fight against biothreats and climate change.
I have already written about the primacy of AI-driven bioinformatics in tackling covid-19, in areas such as reverse vaccinology, computational predictions of protein structures, and epidemiological modelling (e.g. ‘Lessons from a Pandemic: 1. Digital collaboration and preparedness’).
I am now excited to share with you some dispatches from another critical fight, that of tackling climate change. While novel materials and production techniques have all greatly contributed, it is specifically the application of machine learning (ML), a subset of AI, which is currently greatly accelerating the development of commercially viable energy from thermonuclear nuclear fusion.
The sun shone on the Severn
It is April 1982. A British naval task force is sailing to the Falklands. Guinon Bluford becomes NASA’s first African American astronaut. Four-star petrol (remember?) is 36p per litre. Meanwhile on the south bank of the river Severn, a lean teenage punk is toiling on his pre-university research work experience at CEGB’s Berkeley Nuclear Laboratories.
I’d configure banks of autoclaves, monitor various readings and feed these into a creaky mainframe that commanded its own clean room. Our Magnox device at Berkeley was a first generation gas-cooled reactor that used uranium as fuel, with graphite as the moderator and carbon dioxide for cooling. The name referred to the magnesium-aluminium alloy cladding around its fuel rods. The oxidation and spallation of these fuel rods was the subject of my first ever research project and my first ever proper job.
But Magnox reactors were already on the way out. At lunchtime, inbetween the latest news from the Falklands and complaints about the catering, the wise and the good at BNL would sometimes discuss thermonuclear fusion with due reverence. Its spell was addictive. When my then boss assured me that I’d see a commercial fusion reactor on the bank of the river Severn within my lifetime, I had no reason to disbelieve him.
Thermonuclear fusion is simple to explain. Push two atomic nuclei together and, hey presto!, they sometimes fuse to form a larger nucleus, while releasing a huge amount of energy in the process. This is what happens on a massive scale within the cores of stars, including our own sun.
While simple to explain, fusion is incredibly hard and enormously expensive to control on Earth. Pushing nuclei together requires the creation of an electrically-charged gas called plasma, which then needs to be held captive at over 100 million °C and colossal density, typically by a magnetic confinement device, such as a tokamak. In these conditions, electrons leave their shells and form a boiling broth with naked, positively charged nuclei. When the conditions are right, the strong nuclear force between protons from two hydrogen isotopes, deuterium and tritium, overcomes their natural electrostatic repulsion and allows them to fuse, releasing a high energy neutron.
Researchers have produced such controlled fusion reactions for decades. The elusive goal has always been to achieve the reliable, controlled and continuous operation of a sustained fusion chain reaction. And, critically, one which produces net energy. This goal has consistently proven a rolling decade away.
Yet now, something is starting to feel truly different. Both scientists and investors are reporting game-changing progress. Now as they predict first commercial thermonuclear fusion reactors by the end of this decade, it’s without their tongues firmly in cheek or the usual sniggering in the back rows. So what has changed? Certainly not the laws of physics. What has changed is that the massive number crunching required to effectively design, model, test and operate thermonuclear fusion reactors is now made possible by new generation, deep machine learning supercomputing.
Lighting the safe fuse
In the 1990s, the Joint European Torus (JET) tokamak in Oxfordshire delivered 16 million watts of output energy for less than a second. Unfortunately, that still only accounted for only 65% percent of the input energy. Misery, according to the Micawber Principle. However, many reactor tweaks were tested and the international consortium behind JET, armed with new practical experience, went on to form the core of the International Thermonuclear Experimental Reactor (ITER) project.
ITER in Saint-Paul-lès-Durance, southern France, is now the world's largest fusion reactor. Its goal is to produce 500 million watts of power, a commercially viable scale, outputting ten times its input energy (i.e. a Q factor of 10 [i]), albeit in short bursts. Planned result, happiness!
But the ITER project is not without significant problems. It is now years behind schedule and its build cost has tripled to about $22 billion. As a result, its design had to be simplified. Some scientists now even question whether its ambition to deliver “sustained burn”, a fusion chain reaction that could be kept going indefinitely, is at all possible given its downscaled design.
While ITER splutters on, its successor, DEMO, is already on the drawing board for 2033 and is expected to produce 2,000 million watts output, with 80 million watts of input power (Q=25). And further into the future still, DEMO’s successor, PROTO, originally planned for around 2050, would be the European Commission’s first prototype commercial power station. However, given the recent advances, it is possible that these projects could now be brought forward, or even collapsed into one combined DEMO/PROTO project.
The ITER in southern France, with a brave worker shown for scale.
Thermonuclear fusion is safe and clean. Plasma needs to be tightly contained and controlled for a fusion reaction to occur in the first place. So as soon as an anomaly occurs, the reaction simply stops. Unlike fission reactors, there is no risk of a core meltdown. And unlike fission, fusion does not process radioactive fuel such as enriched uranium, which produces dangerous waste. Fusion is typically fed with hydrogen and lithium [ii], and produces only helium and some energetic neutrons.
Fusion reactors can also have a relatively small footprint. This makes it feasible for compact fusion reactors (CFRs) to be embedded into city and community scale smart microgrids. They could even be portable. The US Navy is already working on CFRs as potential next generation powerplants for their vessels. It has filed a patent for a fusion device, which instead of superconducting magnets found in traditional fusion reactors, is based on an "accelerated vibration and/or accelerated spin" magnetic containment design. This uses conical dynamic fusors that spin at extremely high speeds to produce a sustained, concentrated magnetic flux to contain the plasma.
Money is pouring into such research. Nearly thirty hi-tech billionaires such as Jeff Bezos and Bill Gates are behind Breakthrough Energy Ventures, which funds nuclear fusion and other clean energy innovations. Last year, the UK government confirmed an £200m investment to attain grid electricity generation from fusion by 2040. Scientists at UKAEA’s Culham Centre for Fusion Energy near Oxford are working on a detailed design for the Spherical Tokamak for Energy Production (STEP) to deliver this. This initial funding covers their five year concept design phase.
Advanced materials and high temperature superconductors, have allowed tokamak and stellarator designs to greatly improve. New methods of construction have increased their performance and made their production much more affordable. It is now feasible for private companies to compete in the development of fusion. Indeed, several startups are already working on cheap fusion energy with the aim of delivering to the grid by 2030. The real game changer has been the growing availability of powerful cloud supercomputing combined with sophisticated machine learning.
Daniel Kammen, professor of Energy at UC Berkeley, announced in a recent virtual workshop on Tackling Climate Change with Machine Learning, that this transformative computing power has translated the concept of ubiquitous fusion energy from being in "someone else's lifetime to a next-generation project". He added that, "There are now people who are projecting small-trial fusion plants that couldn't have been done before without higher computing."
Modelling the sun
Futurologists at McKinsey predict that AI could add $13trn to the global economy by 2030, comparable to the total national output of China. PwC gives an ever bolder prediction of $16trn. Whatever its economic contribution, AI is revolutionising many aspects of our lives. Machine learning already produces code to recognise faces, understand natural language, steer autonomous vehicles, review your radiology scans, answer your emails and restock your refrigerator. Now it controls thermonuclear fusion here on Earth.
Up to now, the principal limiting factor in fusion reactor design has been its dynamic complexity. Dozens of parameters need to be varied to produce a candidate reactor design, for which predictive simulations then need to be run to model the complex and inherently unstable behaviour of plasma within it. These design and behavioural models often took weeks if not months to produce and validate.
Now, collaborations with leading AI actors are beginning to hugely accelerate this work, reducing such tasks to a few hours. Vancouver-based General Fusion is for example working with Microsoft, while California’s TAE Technologies have teamed up with Alphabet’s DeepMind, who recently publically demonstrated AI’s potential by its system beating one of the world’s best players at Go, the traditional board game. Specialist ML is now central to fusion reactor research.
To clarify, machine learning (ML) is defined as a specific subtype of an AI application. The underlying ML algorithm needs first to be fed with sufficient volume, quality and variety of training data to allow it to develop its internal heuristic.
A pre-configured AI application can, say, control a smart energy grid or a city traffic system. But given exactly the same parameters, the system will in perpetuity make the same interventions. It is only when the application is designed to review the outcome of its interventions and improve, continuously learning and adapting through a feedback cycle, does it become a true ML system. By definition then, not all forms of AI are examples of machine learning.
ML has the inherent ability to identify patterns and correlations, and predict outcomes with rapidly increasing accuracy. It can collate data from a multitude of individual digital sensors in real-time to track the progress of the reaction against its own prediction, continuously refining its algorithm.
Artificial neural network (ANN) computing, inspired by biology, is adaptive and learns to make its own distinctions, without being preset with an extensive task related rule base. It can support the prediction of hugely complex and non-linear reactor behaviour, such as plasma disruptions, with unprecedented accuracy. Actual reactor time and expense are thus saved by simulating many reactor configurations, to identify the most promising one to physically test.
An example of such a deep learning application for modelling plasma disruptions is the Fusion Recurrent Neural Network (FRNN), run by the US Department of Energy's Princeton Plasma Physics Laboratory (PPPL), Princeton University and Julian Kates-Harbeck, a physics Ph.D. student from Harvard University [iii]. The FRNN application was developed using the Google Tensorflow framework and trained on experimental data from the largest tokamaks in the USA (DIII-D) and the world (Joint European Torus, JET). It has learned to reliably predict deleterious plasma disruption events within 30 milliseconds prior to occurrence, which is sufficient warning to microadjust the reactor configuration and mitigate the disruption effect. It is currently being deployed into ITER.
The FRNN deep learning system demonstrates how hugely complex, high-dimensional data can be predictively modelled in real time, to effect the design and operational adjustments needed to control thermonuclear reactions reliably.
“This research opens a promising new chapter in the effort to bring unlimited energy to Earth,” claims PPPL Director Steve Cowley. “Artificial intelligence is exploding across the sciences and now it’s beginning to contribute to the worldwide quest for fusion power.”
In summary
UN’s Intergovernmental Panel on Climate Change (IPCC) had in October 2018 published its famous Special Report
[iv]. It set the global carbon dioxide emissions reduction target of 45% by 2030 to keep the global temperature rise below 1.5°C, and the target of net-zero emissions by 2050.
The covid-19 pandemic has unexpectedly helped in this ambition, but its positive effect on the environment is likely to be short lived. We need a rapid, global decarbonisation of the energy sector. This includes the deployment of wind and solar generation on a massive scale, and the rapid innovation in energy storage solutions.
It could therefore be argued that the $-billions planned as public and private investment in fusion should instead be decanted towards this. Indeed, the struggling ITER is an unhelpful reminder of how elusive commercially viable fusion energy still remains.
Yet I’d like to still believe my old BNL boss. As the quote on the cover of IPCC’s Special Report sagely says, “Pour ce qui est de l’avenir, il ne s’agit pas de le prévoir, mais de le rendre possible“ [v], or in my loose translation, “As far as the future is concerned, it is not a question of foreseeing it, but of making it possible”.
The exponential rise in the applied performance of deep machine learning could soon make thermonuclear fusion viable. I may yet live to see that commercial fusion reactor on the bank of the river Severn.
[i] We have by now during the covid-19 pandemic all mastered the R factor in epidemiology, i.e. the reproductive value, or how many people on average will be infected for every one person who has the disease. There is a similarly critical factor in thermonuclear fusion, Q, the fusion energy gain factor, which is the ratio of fusion power produced in the reactor to the input power required to run it. So when Q=1, the energy produced by the fusion reaction exactly equals the input energy required to sustain it. Pay attention at the back!
[ii] Yes, lithium is currently a bottleneck resource, with lithium-ion batteries needed to power most electronic devices and electric vehicles, and to support large grid scale and home battery storage. Current lithium extraction methods in places such as the Salar de Uyuni salt plain in Bolivia, which holds up to 70% of known global lithium reserves, take months and have a recovery performance of only 30%. Thankfully, new technologies are currently being trialled to vastly increase lithium production, such as the Energy Exploration Technologies’ (EnergyX) nanoparticle membrane that has a recovery performance of 90% within days.
[iii] Kates-Harbeck, J., Svyatkovskiy, A., Tang, W., “Predicting disruptive instabilities in controlled fusion plasmas through deep learning”, Nature 568, 17 April 2019
[iv] You can download the IPCC report and other materials from https://www.ipcc.ch/sr15/download/
[v] From Antoine de Saint Exupéry, Citadelle, 1948
Dr Piotr Ney is an energetic promoter of innovation, digital transformation, customer and operational excellence, and sustainability, with some thirty five years of change leadership, consultancy and senior executive experience. He has an MBA in International Business and a PhD in Economics and Management, lectures part-time in Innovation Management and Disruptive Strategy and is a popular speaker at global business events. Piotr works in London and internationally as an independent consultant and educator.
Copyright © 2020 by Piotr Ney. All rights reserved. No part of this work may be published, reproduced or transmitted in any form or by any means, electronic, mechanical, photocopying, recording, or otherwise, without prior written permission of the author.
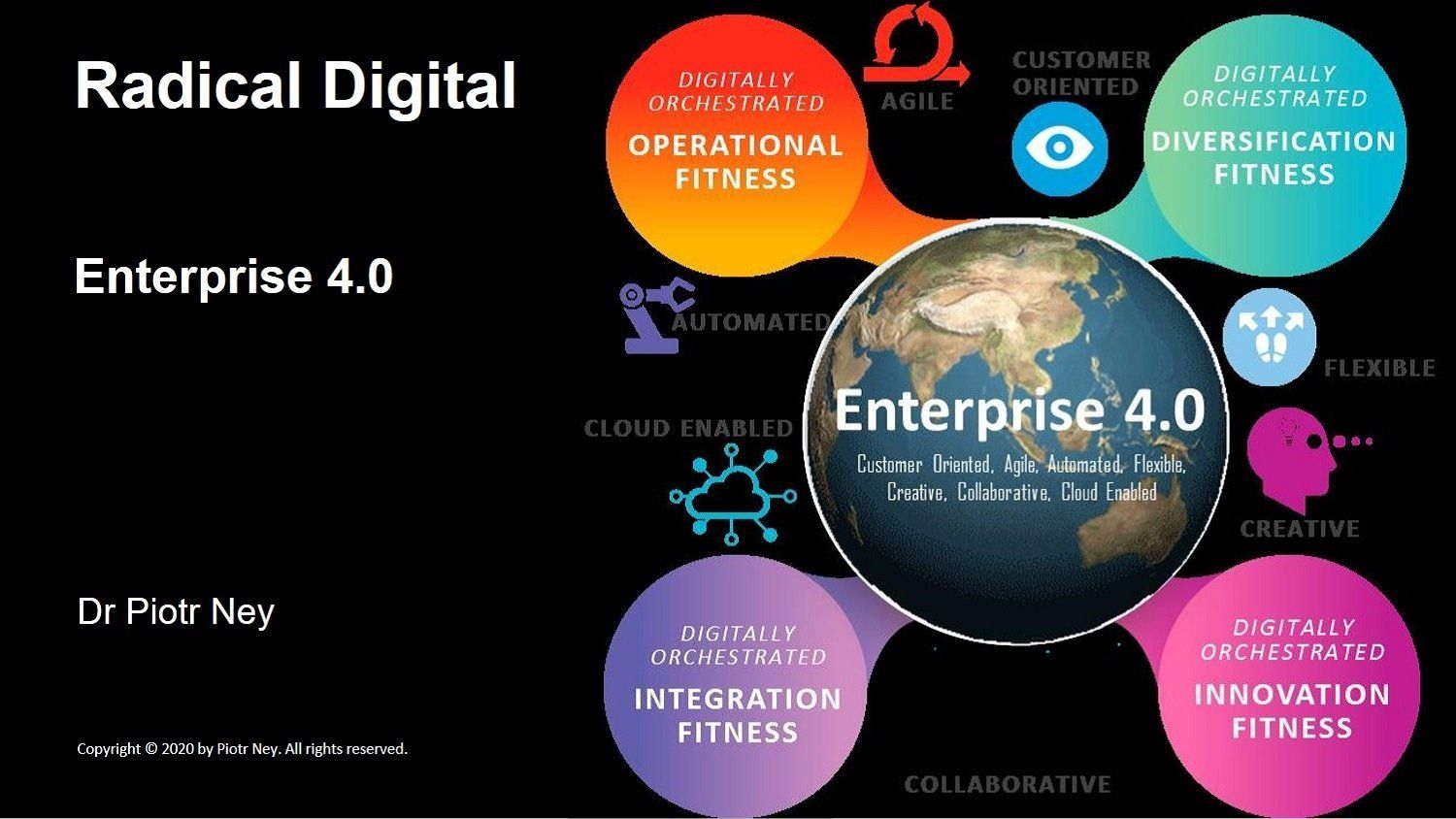